Image Processing: Real-time image dehazing clarifies digital images
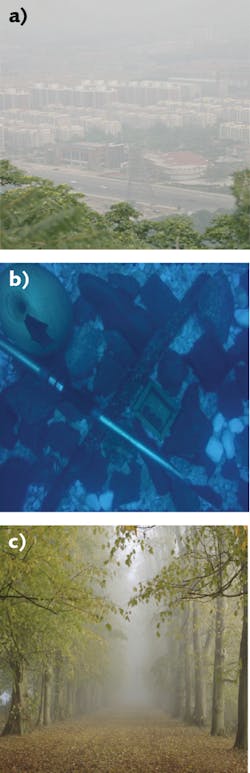
With the rise of digital cameras both in the consumer market and in various sensing systems, the haze-removal of outdoor images is gaining increasing attention. Image dehazing has taken by storm numerous significant scientific fields of applications such as astronomy, medical sciences, remote sensing, surveillance, web mapping, land-use planning, agronomy, archaeology, and environmental studies.
Visual data is the most crucial data comprehended and analyzed by the human brain. About one-third of the cortical area in the human brain is dedicated to analysis of visual data. As a result, image clarity is of uttermost importance for numerous imaging tasks. Often, in practice the light reflected from a subject is scattered by the atmosphere before it reaches the camera.1 This scattering of light results from suspended particles—that is, aerosols such as mist, dust, and fumes that deflect the light from its primary course of propagation.
In vehicular systems, cameras must generate clear images even in bad weather conditions. Because mist and air particles limit the ability to recognize other vehicles, traffic signs, and pedestrians, dehazing is an indispensable requirement in the consumer devices to acquire high-quality images.2 Specifically in the case of remote sensing, this process results in substantial loss of contrast and color of the images. Such images often lack visual vividness and appeal, and moreover, they hinder further image-processing tasks due to poor visibility.
The process of image dehazing serves to improvise aesthetic quality, contrast, and the quality of image information in computer-vision applications and data collection. Dehazing is vital for many computer-vision algorithms such as remote sensing, intelligent vehicle control, underwater image dehazing, object recognition, and surveillance.3, 4
To tackle these problems, a plethora of methods has been documented in literature. Current work on image dehazing consists of image-enhancement-based, image-fusion-based, and image-restoration methods. Some of the current state-of-the-art image dehazing methods are histogram-equalization-based methods, transform-based methods, fusion with multispectral images, and methods based on prior knowledge of the scenes.
Haze has two undermining effects on image: it reduces the image contrast and, as well, adds an additive component to the image called airlight. This article presents a basic idea of image dehazing using a nonlocal (NL) image dehazing tool, which can recover a haze-free image by enhancing the visibility of the scene and correcting color shift.5, 6
Nonlocal image dehazing
To present a vivid depiction of image dehazing, a diverse hazy dataset is chosen here—that is, a city view, a forest view, and an underwater turbid dataset (see Fig. 1). It can be seen that these images show reduced color and contrast, limited object recognition, and visible artifacts. The source images have been acquired from the following two links:
As stated above, haze degrades image visibility, limits color contrast, and forms an additive ambient light component in outdoor images. The degradation of images due to haze is directly proportional to the distance from the sensor (camera) as the magnitude of light increases and radiance of image decreases. Due to this principle, hazy images can be modeled as a combination of global airlight and haze-free pixels.
Berman et al. proposed a NL image-dehazing solution based on NL priors, in contrast to existing local prior methods. This method, being global in nature, does not divide the image on the basis of patches.7 The NL dehazing algorithm follows the hypothesis that colors in a haze-free image can be well approximated by a few hundred discrete colors that form tightly spaced clusters in red-green-blue (RGB) space.
The approach is based on the primary observation that, in a given cluster, the image pixels are often NL—that is, they are positioned at varied distances from the camera while being spread over the entire image plane. Under the influence of haze, these varying distance values translate into distinct transmission coefficients in the optical domain. This leads to the understanding that each cluster in a haze-free image becomes a line in RGB space called a haze line. The authors in Ref. 7 recovered the haze-free lines while obtaining distance maps as well. This is performed linearly on the image matrix, is deterministic in nature, and requires no training.
Image dehazing methodology and experiments
Using the diverse dataset (city view, forest, and underwater image) with haze, the NL image dehazing algorithm was used to generate haze-free images for increased visual perception (see Fig. 2). The input dataset was processed using Matlab R2015b software via grayscale images measuring 512 × 512 pixels. In the first step, the image is assumed to be a combination of haze-free image and ambient light. The image pixels are then clustered together to form haze lines and transmission coefficients and maximum radii are obtained for these haze lines. A haze-free image is obtained by regularizing and minimizing the ambient light.The results have been evaluated using subjective visual analysis as well as quantitative approaches. To quantitatively evaluate the haze-free images, peak signal-to-noise ratio (PSNR) and structural similarity (SSIM) were used. These metrics quantify signal strength, the amount of feature preservation, and recovery of structural features obtained in the haze-free image.
It can be seen from the Figure 2 results that the visual quality of the images is fairly high and allow an extended degree of visual comprehension. Although the resulting radiance in the haze-free images is slightly less than in the original images, the information content is well preserved. The resultant images have higher dynamic range without any color distortion; the NL dehazing algorithm efficiently dehazes images without compromising on significant image features like edges, contours, and details.
These software algorithms can be further advanced for video dehazing and real-time image-dehazing problems. The process of dehazing goes through scene depth estimation, which can also be used in other applications. Dehazing is vital for many computer-vision algorithms used in remote sensing, agricultural monitoring, intelligent vehicles, object recognition, and surveillance that assume the hazy image as original scene radiance and hence suffer from bias. With some experimentation, images obtained from various remote sensors and traffic-monitoring cameras can be readily processed to dehaze the images.
REFERENCES
1. W. Wang and X. Yuan, IEEE/CAA J. Automatica Sinica (2017); doi:10.1109/jas.2017.7510532.
2. C. O. Ancuti and C. Ancuti, IEEE Trans. Image Process., 22, 8, 3271–3282 (2013).
3. X. Wang et al., Int. J. Agric. Biol. Eng., 11, 2, 170–176 (2018).
4. S. Salazar-Colores et al., EURASIP J. Image Video Process., 1, 66 (2019).
5. T. Dong et al., Sensors, 19, 7, 1593 (2019).
6. S. Shao et al., Math. Problems Eng., 4131378 (2019).
7. D. Berman and S. Avidan, “Non-local image dehazing,” Proc. IEEE Int. Conf. Comput. Vis., 1674–1682 (2016).
8. S. Serikawa and H. Lu, Comput. Elect. Eng., 40, 1, 41–50 (2014).
Ayush Dogra | Assistant Professor - Senior Grade, Chitkara University Institute of Engineering and Technology
Dr. Ayush Dogra is an assistant professor (research) - senior grade at Chitkara University Institute of Engineering and Technology (Chitkara University; Punjab, India).
Bhawna Goyal | Assistant Professor, UCRD and ECE departments at Chandigarh University
Dr. Bhawna Goyal is an assistant professor in the UCRD and ECE department at Chandigarh University (Punjab, India), and in the Faculty of Engineering at Sohar University (Sohar, Oman).
Paras Chawla | Professor, Department of Electronics and Communications at Chandigarh University
Paras Chawla is a professor in the Department of Electronics and Communications at Chandigarh University (Punjab, India).
Apoorav Maulik Sharma | Research Fellow, Department of Electronics & Communication Engineering (ECE)
Apoorav Maulik Sharma is a research fellow in the Department of Electronics & Communication Engineering (ECE) in the University Institute of Engineering & Technology (UIET) at Panjab University (Chandigarh, India).
Sanjeev Kumar | Senior Scientist, CSIR-CSIO
Sanjeev Kumar is a senior scientist at the CSIR-Central Scientific Instruments Organisation (CSIO), Chandigarh, India.