Superresolution Fluorescence Imaging: Laptop system produces superresolution fluorescence imagery
About a year ago, we asked whether it might be possible to significantly boost the performance of an optical microscope without altering its hardware or design or requiring user-specified postprocessing. This year, we ask the same question, with an important change: the performance boost involves turning low-resolution fluorescence images into the types of subcellular superresolution images that were the subject of the 2014 Nobel Prize in Chemistry.
Is that possible? The answer, of course, is the same as it was last year: Yes. What’s more, it’s possible for such a system to overcome some limitations of super-imaging in the process.
Researchers at the University of California Los Angeles (UCLA), led by professor Aydogan Ozcan, associate director of the California NanoSystems Institute, proved it by using an off-the-shelf laptop computer (similar to a standard gaming system). In a fraction of a second, the laptop produced superresolution images previously produced only by specialized equipment requiring specialized skill to operate. But the new system is easily accessible by scientists without such expertise, according to postdoctoral scholar Yair Rivenson, co-first author of a paper describing the work.1
The technique’s data-driven approach requires no numerical modeling or estimation of a point-spread function. Instead, it uses a type of artificial intelligence (AI) called deep learning, whereby machines “learn” through exposure to data patterns. This is the engine for transforming microphotographs generated by simple, inexpensive microscopy systems into images with superresolution detail just like those generated by highly complex and costly instruments. Like much of the work that Ozcan’s group does, the new development “democratizes” sophisticated capabilities. The inexpensive, easy-to-use deep-learning setup makes superresolution imaging accessible to researchers without access to expensive, high-end equipment, and thus will no doubt facilitate discovery.
Teaching intelligence
As part of their experiments, the researchers used thousands of microphotographs of cell and tissue samples taken by five types of fluorescence microscopes. They input the images into their computer in matched pairs of low- and superresolution depictions of the same sample.
The computer learned from those images using a “generative adversarial network,” an AI model in which two algorithms compete. One algorithm works to produce computer-generated superresolution output from a low-resolution input image. The second algorithm attempts to differentiate between the resulting computer-generated image and its superresolution counterpart produced by an advanced microscopy system.
Each type of subject requires only one such “training”—after that, the network can transform unfamiliar low-resolution images to achieve the level of detail produced by a superresolution microscope. The study demonstrated that contrast and depth of field were also improved.
In the study, the system transformed wide-field images acquired with low-numerical-aperture (NA) objectives into images matching the resolution of images captured using high-NA objectives (see figure). It also accomplished cross-modality conversions, converting confocal images to match the resolution of images produced by a stimulated emission depletion (STED) microscope, for instance, and using standard total internal reflection fluorescence (TIRF) microscopy imagery to achieve results obtained with TIRF-based structured illumination microscopy.
Naturally, the approach avoids subjecting new samples to the intense light used in standard superresolution microscopy, which can alter the behavior of cells, or damage or kill them. And in the study, it outperformed other resolution-enhancement algorithms.
“Our system learns various types of transformations that you cannot model because they are random in some sense, or very difficult to measure, enabling us to enhance microscopy images at a scale that is unprecedented,” says graduate student Hongda Wang, the other co-first author of the study.
Honor-worthy
It’s work like this that got Ozcan elected a fellow of the National Academy of Inventors in December 2018. The honor is given to academic inventors demonstrating “a prolific spirit of innovation in creating or facilitating outstanding inventions that have made a tangible impact on quality of life, economic development, and welfare of society.”
The month prior, he was elected a fellow of the American Association for the Advancement of Science. That honor recognizes major efforts that have advanced science or its applications either scientifically or socially. It cited Ozcan for his “distinguished contributions to photonics research and technology development on computational imaging, sensing, and diagnostics systems, impacting telemedicine and global health applications.”
NAI highlighted Ozcan’s pioneering and high-impact inventions (in mobile health, telemedicine, microscopy, sensing, and diagnostics) with the potential to dramatically increase access to medical technologies in resource-limited settings and developing countries. His company Cellmic’s smartphone-based diagnostic test reader facilitates medical field work in remote locations, providing ease of use, speedy test results, and linkage to a central database.
REFERENCE
1. H. Wang et al., Nat. Methods, 16, 103–110 (2019)
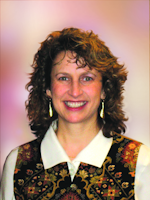
Barbara Gefvert | Editor-in-Chief, BioOptics World (2008-2020)
Barbara G. Gefvert has been a science and technology editor and writer since 1987, and served as editor in chief on multiple publications, including Sensors magazine for nearly a decade.