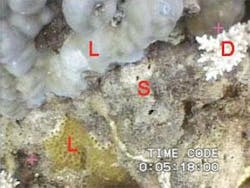
Vision systems excel at locating or sorting man-made objects, which usually have sharp edges or predefined shapes that ease the requirements and up the success rates of image-processsing algorithms. Natural objects, on the other hand, tend to have great variability and subtler distinguishing characteristics. But researchers at the National Institute of Physics at the University of the Philippines (Quezon City, Philippines) are using a neural-network-based vision system to classify a very variable object indeed-coral.
The scheme arose from the need to monitor coral reefs quickly and frequently to determine whether they are healthy, at risk, damaged, or recovering. Potential sources of damage include storms, trawling, and dynamite fishing. While humans can classify coral by species to an accuracy of 90% ±8% in video point-sampling, and can determine whether the coral is living or dead to that accuracy as well, the process is slow. In response, the Philippine researchers have become the first to perform close-up automatic image recognition of coral reefs.
The colors and textures of corals vary enormously, and they change appearance based on the viewer’s perspective. For this reason, the researchers decided to restrict their classification to three categories: live coral, dead coral, and sand or rubble (they are now working on expanding the number of categories). The researchers chose the Matlab neural-network toolbox developed by MathWorks (Natick, MA); while the Philippine researchers designed the network architecture, training rates, and other parameters, no other customization was done to the toolbox, according to Caesar Saloma, one of the researchers.
Accuracy from ambiguity
The neural network concentrated on two coral features-color and texture. For color, the image was converted into two separate color spaces. The first was a 2-D color space in which color information is separate from intensity-necessary for use with images from underwater videos of varying brightness. Color histograms were compiled for four major coral color groups: red-orange-yellow-brown, blue, green, and white-gray. The second color space was based on hue (the difference between the color and a pure color such as red), saturation, and brightness. The texture operator, called “local binary patterns,” gets statistical information from spatial relationships of pixels. In the experiment, the neural-net classifier was compared against a simpler two-step decision-tree classifier.
For test, preclassified image frames were taken from an underwater video of a small portion of Australia’s Great Barrier Reef and smaller segments cut from the images for test, resulting in 185 images-98 of live coral, 43 of dead coral, and 44 of sand (see figure). The neural-net classifier achieved 86.5% and 82.7% recognition rates for analyses based on the two different color spaces, with the 2-D color space achieving the higher rate. The two-step classifier achieved 79.7% and 79.3% recognition rates.The researchers note that the neural net will require retraining for videos taken at different depths, because the blue-green tint of seawater changes the color properties. Underwater lighting could also help.
“At the moment we can classify living and dead coral and sand,” says Saloma. “However, it is also important to measure the amount of algae cover because it is an indicator of the reef’s robustness and resiliency. In essence, the applicability of the current performance of our system would depend on the problem of the marine scientist. If a need for rapid assessment of living and nonliving areas is desired, then our system would suffice.” Increasing the number of categories analyzed by the neural net should boost the classification accuracy, he notes.
REFERENCE
1. Ma. Sheila Angeli C. Marcos et al., Optics Express13(22) (Oct. 31, 2005).
About the Author
John Wallace
Senior Technical Editor (1998-2022)
John Wallace was with Laser Focus World for nearly 25 years, retiring in late June 2022. He obtained a bachelor's degree in mechanical engineering and physics at Rutgers University and a master's in optical engineering at the University of Rochester. Before becoming an editor, John worked as an engineer at RCA, Exxon, Eastman Kodak, and GCA Corporation.