Machine-vision systems that incorporate faster processors and better software algorithms are helping researchers monitor and commercial fisheries harvest numerous aquatic species. For example, in the Columbia River Basin in the U.S. Northwest, counting the types and numbers of migrating fish is an important step in managing the protection of endangered species and in bringing fish to market.
Currently, fish populations are monitored manually by reviewing 24-hour, time-lapse videotapes made during the migration season as the fish climb ladders over manmade dams. In some cases, videotaping is not considered reliable; therefore, a trained biologist may identify and count the fish. Counting fish in their native underwater surroundings with a camera poses many challenges: electronics do not work well in wet environments; visual contrast is reduced in underwater settings by bubbles, turbidity, sediment, and debris; and fish come in all shapes and sizes. Complicating the counting and classifying process is the fact that fish rarely stop in perfect profile, offering twisted, rotated, and moving targets for image-processing algorithms.
These challenges are being addressed at the Prosser Dam on the Yakima River tributary of the Columbia, where Agris-Schoen Vision Systems (Merrifield, VA) and scientists from the Brigham Young University (Provo, UT) teamed to devise an automatic fish-counting and classification system. A near-infrared CCD camera, frame grabber, and custom image-analysis routine count the fish through a window as they climb the ladder around the dam, collecting data on type, length, and other physical characteristics.
Many denizens of the deep do not move and therefore cannot be imaged in situ as they pass a camera-deep-sea scallops, for instance. Between July and August each year, the U.S. National Oceanic and Atmospheric Administration Fisheries Service conducts surveys to determine the abundance and size distribution of deep-sea scallops in areas along the U.S. Northeast coast. To do this, sample height measurements from 125,000 scallops are taken from approximately 500 randomly selected locations using an 8 ft scallop dredge towed at 3.5 knots for 15 minutes. To increase the accuracy and speed of these measurements, Woods Hole Laboratory (Woods Hole, MA) is developing a prototype machine-vision system designed by Compass Technical Consulting (Rochester, NY). The prototype system consists of a standard conveyor modified to include an electroluminescent backlight and a shroud to house the FireWire camera and laptop PC.
One challenge was the range of scallop sizes and geometries to be inspected. Sea scallops, Icelandic scallops, and calico scallops can range from a few millimeters to 200 mm in height. All three species are loaded in any orientation and located anywhere across the belt. To solve this problem, a “soft triggering” method was developed using regions of interest in the camera’s field of view. As images are captured continuously at 30 frames/s, each image is inspected for the presence of a scallop and the image processed when a scallop is detected.
Into the hopper
Beyond research, machine vision supports the bottom line onboard commercial fishing vessels. To avoid spoilage, these vessels carry facilities for processing their catch. Human labor is at a premium, so commercial fishing concerns install automation whenever possible. One task that has resisted automation, however, is ensuring that only high-quality fish go into the filleting machines and that they go into the machines head first; otherwise, they would be ruined in the machine.
Pisces VMK (Wells, MI), working with General Vision (Petaluma, CA), developed a system to help automate the inspection process using machine vision and artificial intelligence. Since artificial-intelligence solutions “learn” by experience, the system is better able to find a correct match as it gains experience with different shapes, and its ability to categorize new targets approaches 100%.
The system easily keeps up with a processing line running at 360 fish per minute, but can go faster. This performance allows the line to run faster than was possible with human operators, so the boat fills its cargo hold in five days instead of seven-giving more meaning to the expression, “fresh from the sea.”
About the Author
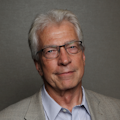
Conard Holton
Conard Holton has 25 years of science and technology editing and writing experience. He was formerly a staff member and consultant for government agencies such as the New York State Energy Research and Development Authority and the International Atomic Energy Agency, and engineering companies such as Bechtel. He joined Laser Focus World in 1997 as senior editor, becoming editor in chief of WDM Solutions, which he founded in 1999. In 2003 he joined Vision Systems Design as editor in chief, while continuing as contributing editor at Laser Focus World. Conard became editor in chief of Laser Focus World in August 2011, a role in which he served through August 2018. He then served as Editor at Large for Laser Focus World and Co-Chair of the Lasers & Photonics Marketplace Seminar from August 2018 through January 2022. He received his B.A. from the University of Pennsylvania, with additional studies at the Colorado School of Mines and Medill School of Journalism at Northwestern University.