In a 1958 paper in Psychological Review, Frank Rosenblatt described the first neural network capable of learning through a simple reinforcement rule. The network learned by changing the weight applied to the input signal by an amount proportional to the difference between the desired and actual outputs. Although the neural network had only two neuron layers and accepted binary input and output values, Rosenblatt showed that the network could solve basic AND or OR logic operations.
Marvin Minsky and Seymour Papert of the Massachusetts Institute of Technology (Cambridge, MA) extended the concept in 1969 to a neural network that consisted of an input layer (where data were fed into the network), one or more hidden layers (representing the internal neurons in the brain), and an output layer that produced the result of the computation. The ability of the neural network to produce a desired result depended on the number of neurons in the network, how the neurons were connected, and the values of the input weights.
Since the introduction of feed-forward networks, such as the multilayer perceptron model, researchers have developed other models in which the neurons are connected in ways designed to better model specific applications such as logical operation, pattern classification, and speech recognition.
“Electronic neural networks accomplish a particular type of learning and decision-making process similar to that of the human brain,” says Fred Turek, COO of FSI Technologies (Lombard, IL). “Indeed, the human brain routinely does certain things no computer has ever come close to doing. Fundamentally, these tasks rely on the ability to learn and make decisions in multivariable processes where, strictly speaking, the information is incomplete and the equation is complex and unknown. While many define the word “decision” as determining a specific course of action, another type of decision of more interest in machine vision is classification.”
Now playing in machine vision
Because of its usefulness in image classification, neural networking is often integrated in machine-vision hardware and software. For example, 10 years ago Guy Paillet, at General Vision (Petaluma, CA) and Toshi Hori, then president of Pulnix (San Jose, CA) formed an alliance to develop a neural-network-based camera. The camera, ZiCAM, was based around a neuron chip called a zero-instruction-set computer (ZISC) whose concept, invented by Guy Paillet, was jointly developed with an IBM team led by Pascal Tannhof in Paris.
After purchasing Pulnix in 2004, JAI (Glostrup, Denmark) discontinued support of neural-network-based cameras. But General Vision did not abandon the idea and now offers several trainable vision-system configurations based around the technology. One system, the CogniSight4-Iris is a neural-network-based software implementation based around the Iris smart camera from Matrox Imaging (Dorval, QC, Canada).
While the original ZISC processor in the Pulnix camera used 78 neurons/chip, Paillet and Hori recognized the need to incorporate a more powerful version of the device into a camera system. To do so, Hori recently formed GEViCAM (Sunnyvale, CA), a company that will exploit the power of a higher-performance hardware neural network device named CogniMem in a range of Gigabit Ethernet-based cameras.
FSI Machine Vision (Lombard, IL), the North American partner of NeuroCheck (Stuttgart, Germany), has integrated a vision-inspection system for Norcon Systems (Lombard, IL) using an FSI CVS-700 machine-vision system and NeuroCheck neural-network software. This integrated system has been deployed to inspect automotive cartridge filters.
At IBM, a neural-network-based system, this time from Sightech (San Jose, CA) has been deployed to inspect the disk platters used in disk drives. To determine whether platters are missing, crossed, and/or doubled within each cassette slot, no C- or GUI-based programming is required. Instead, the system developer presents the vision system with a number of options that include correct and incorrect platter positioning within the cassette. The system then compiles a database of features in association with other features. After correct and incorrect images are presented, the system compares newly presented images with this database of features.
About the Author
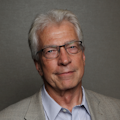
Conard Holton
Conard Holton has 25 years of science and technology editing and writing experience. He was formerly a staff member and consultant for government agencies such as the New York State Energy Research and Development Authority and the International Atomic Energy Agency, and engineering companies such as Bechtel. He joined Laser Focus World in 1997 as senior editor, becoming editor in chief of WDM Solutions, which he founded in 1999. In 2003 he joined Vision Systems Design as editor in chief, while continuing as contributing editor at Laser Focus World. Conard became editor in chief of Laser Focus World in August 2011, a role in which he served through August 2018. He then served as Editor at Large for Laser Focus World and Co-Chair of the Lasers & Photonics Marketplace Seminar from August 2018 through January 2022. He received his B.A. from the University of Pennsylvania, with additional studies at the Colorado School of Mines and Medill School of Journalism at Northwestern University.