Non-line-of-sight (NLOS) imaging has been demonstrated using various computational algorithms at different operational wavelengths. Typically, high-power pulsed light sources and ultrasensitive detectors are used to distinguish strong photons reflected by a visible object from the weak-intensity photons reflected from the hidden portions of the object. Unfortunately, these transient techniques look at photon intensity and transit time only, missing important information used to reconstruct a 3D volume of the hidden object. By using Fermat’s (least-time) optics principle, which states that the path taken by a light ray is the path that can be traversed in the shortest time, researchers at Carnegie Mellon University (CMU; Pittsburgh, PA) and the University of Toronto in Canada have improved NLOS object shape reconstruction using a geometric rather than time/intensity algorithm.
For a hidden object located around the corner from a source/sensor (or obscured by a diffuser), a Fermat flow algorithm is defined wherein the spatial derivative of the Fermat light path is used to determine the depth and normal of a hidden point in the scene. Through numerical fitting and mesh smoothing of depth and normal information for multiple points, the actual surface of the NLOS object is reconstructed. Using a 532 nm, 1 mW pulsed (80 MHz) laser and single-photon avalanche diode (SPAD) detector as well as a time-correlated single-photon counter (TCSPC), the method can reconstruct micrometer- through centimeter-scale objects with a variety of shapes and surface reflectance parameters with an approximate physical difference error of only 2 mm (by comparing reconstructions with CAD meshes when printing some 3D test shapes). By exploiting Fermat’s principle, the researchers are getting close to reconstructing NLOS hidden objects with nearly the same accuracy as objects directly visible to the camera. Reference: S. Xin et al., “A Theory of Fermat Paths for Non-Line-of-Sight Shape Reconstruction,” IEEE/CVF Conference on Computer Vision and Pattern Recognition (CVPR), Long Beach, CA (Jun. 2019).
About the Author
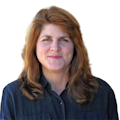
Gail Overton
Senior Editor (2004-2020)
Gail has more than 30 years of engineering, marketing, product management, and editorial experience in the photonics and optical communications industry. Before joining the staff at Laser Focus World in 2004, she held many product management and product marketing roles in the fiber-optics industry, most notably at Hughes (El Segundo, CA), GTE Labs (Waltham, MA), Corning (Corning, NY), Photon Kinetics (Beaverton, OR), and Newport Corporation (Irvine, CA). During her marketing career, Gail published articles in WDM Solutions and Sensors magazine and traveled internationally to conduct product and sales training. Gail received her BS degree in physics, with an emphasis in optics, from San Diego State University in San Diego, CA in May 1986.