Integrated Optics: All-optical neural network can be 100 times faster than electronic versions
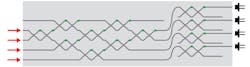
Artificial intelligence (AI), which has been in practical existence since the middle of the last century, has now become central to our vision of a connected, automated society, with the potential to simplify communications between humans and devices, anticipate the needs of humans, and safely drive their vehicles while making moral decisions on what best to do when faced with an accident. The growth of AI, in the form of "deep learning," enabled a computer to beat a master player (the European champion) at the game of Go for the first time in early 2016. Go is considered a much more difficult game than chess.
In deep learning, an algorithm, often realized by an electronics-based artificial neural network (ANN), or a software simulation of a neural network, learns how to master an ambiguous task (such as speech recognition) by observing the task and subsequent results over and over again while modifying the algorithm itself until the task is more or less learned. However, the approach based on electronics is limited by the speed of electronic devices themselves.
Electronic/photonic hybrid ANN prototypes have been created, but such devices are still limited by the speed of the electronics, as well as the ohmic power losses in the electronics.
Now, a group from the Massachusetts Institute of Technology (MIT; Cambridge, MA) and Twitter (also in Cambridge) has developed and tested a prototype of an all-optical ANN that can potentially boost the speed of deep-learning AI by two orders of magnitude, while at the same time reducing the power needed by three orders of magnitude.1
Network of Mach-Zehnder interferometers
Artificial neural networks, either electronic or optical, need to have both linear elements to do matrix multiplication and nonlinear elements to apply a nonlinear "application function." In the MIT/Twitter device design, the linear optical calculations are done using a waveguide-based network consisting of Mach-Zehnder interferometers and phase-shifting elements. The nonlinear activation is done using a nonlinear optical device such as a dye, semiconductor, or graphene saturable absorber or a bistable optical device.
However, in the experimental MIT/Twitter device, which is a first prototype (see Fig. 1), only the linear optical devices were fabricated and tested—the nonlinear operation was done in software. Sometime in the future, nonlinear optics will be integrated into an all-optical ANN, which will then be able to truly realize the advantages of photonics.
The experimental optical ANN contains 56 Mach-Zehnder interferometers and 213 thermo-optic phase shifters. Each interferometer contains two evanescent couplers, along with one internal phase shifter to control the splitting ratio for the interferometer and followed by an external phase shifter to control the relative phase between the interferometer's two outputs. The device's architecture consisted of four layers with four neurons in each layer. The output of each layer was measured using a photodetector array, the nonlinear operation computationally (electronically) applied, and the output then sent into the next layer.
The researchers tested the device by training it to do vowel recognition, using 360 data points generated by 90 people saying four different vowel phonemes. Each data point was translated into a vector that represented the power contained in different audio-frequency bands. The ANN was then trained.
The device correctly identified 138 out of the 180, or 76.7% of the vowel phonemes. A computer model of the device showed a simulated correction of 165 out of 180 cases, or 91.7% (see Fig. 2).In addition to the use of optical nonlinear devices instead of electronic nonlinear computation, future all-optical ANNs can realize a reduction in power by using passive phase-shifters based on nonvolatile phase-change materials, rather than the phase-shifters used in the experiment, each of which required 10 mW of power on average. This and other improvements could lead to a system operation rate of 2m · N2 · 1011 operations/s, where m is the number of layers and N is the number of nodes in the N × N matrix. The researchers also state that, because of the low (in principle, nil) energy requirements of optics, the larger the neural network is, the more it benefits by being optical.
REFERENCE
1. Y. Shen et al., arXiv:1610.02365v1 [physics.optics] (Oct. 7, 2016).
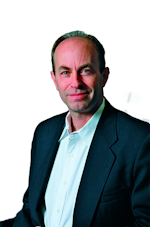
John Wallace | Senior Technical Editor (1998-2022)
John Wallace was with Laser Focus World for nearly 25 years, retiring in late June 2022. He obtained a bachelor's degree in mechanical engineering and physics at Rutgers University and a master's in optical engineering at the University of Rochester. Before becoming an editor, John worked as an engineer at RCA, Exxon, Eastman Kodak, and GCA Corporation.