Neurologists estimate that as much as half the brain is dedicated to visual processing; in that sense, the brain can be regarded as an extension of the eye. In recent years, neurobiologists have been flipping that around and regarding the eye as an extension of the brain. The advance of imaging technology opens up the possibility of identifying anatomical and physiological features of the retina as signatures for degenerative diseases of the central nervous system (CNS). Now, a newly formed company has developed a noninvasive device to identify and quantify functional changes in the eye that can monitor specific CNS disorders.
C. Light Technologies, a startup founded by two researchers at the University of California, Berkeley, images retinal cells to track eye motion. Other eye trackers on the market examine reflections from the cornea to calculate the direction of the gaze. According to C. Light, retinal tracking is more than 100X more accurate than corneal tracking. The increased accuracy allows for precise measurements of the direction, rate, and number of eye motions. Initial research has just demonstrated the effectiveness of the device in identifying the severity of multiple sclerosis.
How to track the retina
Christy Sheehy and Zachary Helft collaborated on eye research when both were at UC Berkeley. Sheehy developed hardware to image the retina rapidly and accurately, while Helft investigated methods to restore degraded vision. During their collaboration at Berkeley, they realized that their technology could be refined to produce a compact device with the potential to provide insight into eye function and, consequently, brain function. They formed C. Light Technologies to pursue that application.
Their device scans a near-IR laser across a 5° × 5° field of view and collects reflected-light images at a 30 Hz frame rate. Images are collected for 10 seconds. During acquisition, eye motions present different portions of the retina to the imaging system, and after acquisition a kind of composite reference map is constructed—a map representing every part of the retina that was imaged during the 10 seconds. Each frame of the image is cut into 16 strips and each section overlaid onto the reference map. Postprocessing then generates a record of eye motion by comparing the position of each strip to its position at the initial acquisition.
In effect, this results in a 480 Hz acquisition rate for position information. That means that each 10-second dataset contains about 5000 measurements of eye position. Depending upon the application, an alternate algorithm can provide about 10,000 measurements over the 10 seconds. The record is analyzed to generate such metrics as the number of eye motions, their frequency, their amplitude, and eye velocity and acceleration.
With their device performance in hand, the question then became: Can these measurements of eye motion be correlated with a specific CNS condition?
Eye, brain, and multiple sclerosis
Sheehy and Helft chose multiple sclerosis (MS) as their first target. The hope is that the eye tracker can monitor the disease and serve as an objective metric to evaluate potential drug therapies. Their initial study compared the eye-motion characteristics of 111 people diagnosed with MS to those of 100 controls. Because MS is characterized by decreased motor control, Sheehy, Helft, and their collaborators hypothesized that MS would have an effect on one of the smallest muscular movements of the human body: microsaccades.
Microsaccades are the little movements made by the human eye even when trying to stay fixated on one spot. For this investigation, subjects were asked to fixate on a corner of a red square. The researchers found people with MS to have more microsaccades than people without, and the MS patients also displayed larger vertical displacements with a smaller peak acceleration. The researchers used two logistic regression models to determine if the eye measurements correlated with other clinical measures of the severity of MS. Using a standard method for evaluating the effectiveness of artificial intelligence processing, their classification demonstrated an 87% area under the receiver operating characteristic curve. The paper concludes that their device “provides a highly accurate, sensitive, and noninvasive retinal eye-tracking device suited for large-scale patient screenings.”
Sheehy says that the company has developed a yet-unpublished feature-based neural network that accurately indicates a “disability score” for MS patients. “We’re poised,” she says, “to surpass the current standard of care by later this year.”
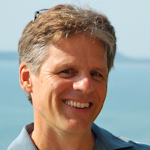
Richard Gaughan | Contributing Writer, BioOptics World
Richard Gaughan is the Owner of Mountain Optical Systems and a contributing writer for BioOptics World.