Optical Coherence Tomography: Innovations in OCT
Given the dramatic contributions of optical coherence tomography (OCT) to biomedicine, it is fitting that the first plenary session at SPIE Photonics West 2019 opened by honoring work in OCT by one of its pioneering developers. The BiOS Hot Topics plenary kicked off with presentation of the 2019 Biophotonics Technology Innovator Award to Stephen Boppart, director of the Center for Optical Molecular Imaging and leader of the Biophotonics Imaging Laboratory at the University of Illinois at Urbana-Champaign. The award particularly recognizes Boppart’s work in developing novel technology for computational OCT and applying it to basic and clinical sciences.
Computational OCT is an evolutionary progression from the original OCT, akin to the development of magnetic resonance imaging (MRI) from nuclear magnetic resonance.1 Computational OCT leverages advances in computing power to address results-degrading limitations of traditional OCT, including the inherent tradeoff between depth-of-field and transverse resolution, the dispersion mismatch of sample and reference paths, and aberrations imposed by optics. Taken together, computational techniques not only improve the quality of OCT imaging, but also boost imaging speed and enable OCT data to provide greater insight. And because they manipulate data, they allow for simpler, lower-cost setups, greater flexibility, and require much less time to produce images. And while many recent developments in OCT have involved computational approaches (including one from Boppart’s group that achieves wavefront correction by combining computational and hardware methods2; see Fig. 1), a number of advances have emerged—even just in the past year—from other types of innovation.
Conditioning monochromatic light for OCT
At SPIE Photonics West 2018, Prof. Dalip Singh Mehta of the Indian Institute of Technology Delhi (India) described a way to improve spatial coherence of a light source while simultaneously maintaining other characteristics necessary for optimal performance.3 OCT performance depends in large part on coherence properties of the system’s light source. Interferometers measure intensity patterns that result from recombining separated parts of an optical beam (sample and reference paths). A path difference of an integral number of wavelengths provides an intensity maximum, while half a wavelength longer or shorter gives an intensity minimum. Two beams will interfere only if they have a well-defined phase difference—that is, if their phases are correlated. The measure of correlation as a function of distance is the coherence length. The intensity variation of two recombined beams—or, equivalently, the fringe contrast—vanishes if the difference between the two path lengths is greater than the coherence length.
OCT uniquely identifies the distance to different surfaces in the object path. For ophthalmological applications, those structures are layers within and beneath the retina. There’s an intensity maximum if the distance to a particular layer, say the retinal pigment epithelium, exactly matches the reference distance. If, however, the surface of the choroid membrane is, say, exactly 30 wavelengths away, then that surface will also provide an intensity maximum—that is, if the coherence length of the source is greater than 30 wavelengths.
To eliminate degeneracy, the traditional practice in OCT is to use a broadband light source. But a broadband source is inherently subject to birefringence effects in the sample. Also, the relatively low power output demands that the source be scanned across the sample point-by-point. It would be far more convenient to acquire a full 2D image in a single shot. Both of those problems could be overcome if the broadband source were replaced by a laser source, but lasers intrinsically have long coherence lengths, which degrades the axial (depth) resolution from the few microns of broadband OCT to several millimeters.
Mehta’s team devised a beam conditioning method that reduces spatial coherence while retaining the monochromaticity and intensity necessary for an ideal OCT source (see Fig. 2). The multistage process starts by expanding the source beam, splitting it in three, and combining those beams on a diffuser at angles of 30°, 0°, and -30°. A condenser lens couples the diffuser light into a vibrating multimode fiber bundle and the output of that fiber bundle is collimated to become the OCT source.
The conditioning introduces spatial, angular, and temporal diversity into the monochromatic beam. Axial resolution is then a function of longitudinal spatial coherence rather than temporal coherence. “With our system,” Mehta says, “we can solve two problems simultaneously: we significantly reduce the speckle effect, leading to uniform illumination, and also decrease the sample interactions using our broad angular frequency spectrum light source.”
Prior work, Mehta says, implicitly assumed that short temporal coherence was necessary for good axial resolution. Then, he says, “our group and some others thought to play with the spatial coherence properties of a monochromatic light source.” The different approach is paying off: Mehta’s group has demonstrated axial resolution of ~4 µm and lateral resolution of ~2 µm.
Learning and quantification
Mehta’s team has since gone on to apply machine learning to OCT tissue analysis and also to leverage machine learning to quantitatively classify damage to human skin inflicted by burns.4 While other groups have worked on quantitative assessment of such damage using OCT, Mehta’s team seems to be the first to report fully automated detection of burn injuries in vivo in humans. The researchers’ objective was to assess surgical margins automatically based on OCT imaging. Working to extract quantitative features from OCT images depicting normal and burned tissues, they created a classifier for machine learning and then applied it to train a linear classification model. Testing of the model revealed 90% specificity and 91.6% sensitivity. Further, the team has developed a deep learning architecture called LightOCT to classify OCT images into diagnostically relevant classes.
Other applications of deep learning have also begun to address OCT imaging as well. For instance, researchers at Shanghai Jiao Tong University (Shanghai, China) have applied this type of artificial intelligence to 3D endoscopic OCT, with a goal of faster procedures.5
Current methods use a data-intensive process to create 3D OCT images by assembling series of 2D images taken sequentially at equal distances. The Shanghai Jiao Tong team used “sparse sampling” to acquire dramatically fewer 2D images (for example, 120 frames vs. 200 in standard OCT), and then applied compressive sensing algorithms to supply missing data to create the 3D depictions. The researchers’ tests confirmed that effective 3D OCT imaging can be done with 40% less experimental data. “After we perform enough experiments to demonstrate that our probe and imaging method are useful for observing malignant features, our technique will be ready for clinical trials,” says team leader Jigang Wu.
REFERENCES
1. Y.-Z. Liu, F. A. South, Y. Xu, P. S. Carney, and S. A. Boppart, Biomed. Opt. Express, 8, 1549–1574 (2017).
2. F. A. South et al., Biomed. Opt. Express, 9, 6, 2562–2574 (2018).
3. D. S. Mehta et al., Proc. SPIE, 10503, 1050305 (2018); https://doi.org/10.1117/12.2290334.
4. N. Singla, V. Srivastava, and D. S. Mehta, Laser Phys. Lett., 15, 2 (2018).
5. J. Wang, Y. Hu, and J. Wu, Appl. Opt., 57, 34, 10056–10061 (2018).
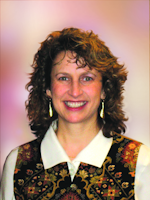
Barbara Gefvert | Editor-in-Chief, BioOptics World (2008-2020)
Barbara G. Gefvert has been a science and technology editor and writer since 1987, and served as editor in chief on multiple publications, including Sensors magazine for nearly a decade.
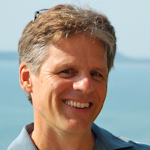
Richard Gaughan | Contributing Writer, BioOptics World
Richard Gaughan is the Owner of Mountain Optical Systems and a contributing writer for BioOptics World.